42 in supervised learning class labels of the training samples are known
6 Types of Supervised Learning You Must Know About in 2022 In Supervised Learning, a machine is trained using 'labeled' data. Datasets are said to be labeled when they contain both input and output parameters. In other words, the data has already been tagged with the correct answer. So, the technique mimics a classroom environment where a student learns in the presence of a supervisor or teacher. Types Of Machine Learning: Supervised Vs Unsupervised Learning Supervised learning is learning with the help of labeled data. The ML algorithms are fed with a training dataset in which for every input data the output is known, to predict future outcomes. This model is highly accurate and fast, but it requires high expertise and time to build. Also, these models require rebuilding if the data changes.
Supervised Learning - an overview | ScienceDirect Topics The procedure of Supervised Learning can be described as the follows: we use x(i) to denote the input variables, and y(i) to denote the output variable. A pair ( x(i), y(i)) is a training example, and the training set that we will use to learn is { ( x(i), y(i) ), i = 1, 2, …, m }. ( i) in the notation is an index into the training set.
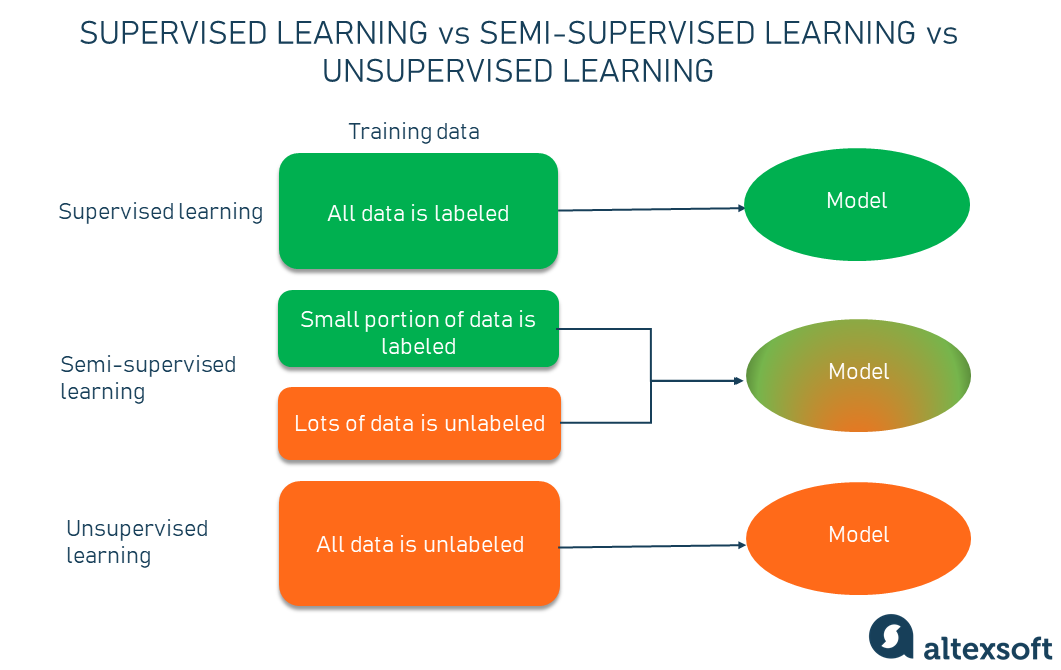
In supervised learning class labels of the training samples are known
Supervised vs Unsupervised Learning: Difference Between Them - Guru99 Unsupervised learning is computationally complex. Use of Data. Supervised learning model uses training data to learn a link between the input and the outputs. Unsupervised learning does not use output data. Accuracy of Results. Highly accurate and trustworthy method. Less accurate and trustworthy method. Prototypical Classifier for Robust Class-Imbalanced Learning In this paper, we address both training set biases simultaneously. As shown in Fig. 1a, it is known that the classifier directly learned on class-imbalanced data is biased towards head classes [8, 32] which results in poor generalization on tail classes.Moreover, using sample loss/confidence produced by biased classifiers fails to detect label noise, because both clean and noisy samples of ... What is Supervised Learning? | TIBCO Software Supervised learning solves known problems and uses a labeled data set to train an algorithm to perform specific tasks. It uses models to predict known outcomes such as "What is the color of the image?" "How many people are in the image?" "What factors are driving fraud or product defects?" etc.
In supervised learning class labels of the training samples are known. Difference Between Classification and Clustering Classification is the process of classifying the data with the help of class labels. On the other hand, Clustering is similar to classification but there are no predefined class labels. Classification is geared with supervised learning. As against, clustering is also known as unsupervised learning. Training sample is provided in classification ... 120 questions with answers in SUPERVISED LEARNING | Science topic Dear N. Janardhan. Supervised learning is a machine learning method distinguished by the use of labelled datasets. The datasets are intended to train or "supervise" computers in properly ... PDF Supervised Learning: Classificaon - fenyolab.org • The known label of test sample is compared with the classified result from the model • Accuracy rate is the percentage of test set samples that are correctly classified by the model • Test set is independent of training set (otherwise over-fing) • If the accuracy is acceptable, use the model to classify new data Semi-Supervised Learning With Label Spreading - Machine Learning Mastery A popular approach to semi-supervised learning is to create a graph that connects examples in the training dataset and propagates known labels through the edges of the graph to label unlabeled examples. An example of this approach to semi-supervised learning is the label spreading algorithm for classification predictive modeling.
Supervised and Unsupervised learning - Dataaspirant Supervised learning is a data mining task of inferring a function from labeled training data .The training data consist of a set of training examples. In supervised learning, each example is a pair consisting of an input object (typically a vector) and the desired output value (also called the supervisory signal ). Supervised and Unsupervised learning - GeeksforGeeks Supervised learning, as the name indicates, has the presence of a supervisor as a teacher. Basically supervised learning is when we teach or train the machine using data that is well labelled. Which means some data is already tagged with the correct answer. Lecture 1: Supervised Learning - Cornell University Let us formalize the supervised machine learning setup. Our training data comes in pairs of inputs ( x, y), where x ∈ R d is the input instance and y its label. The entire training data is denoted as D = { ( x 1, y 1), …, ( x n, y n) } ⊆ R d × C where: R d is the d-dimensional feature space x i is the input vector of the i t h sample Basics of Supervised Learning (Classification) | by Tarun Gupta ... They are namely Learning and Querying phase. The learning phase consists of two components of namely Induction (training) and Deduction (testing). The querying phase is also known as application phase. Let's talk about it in a more formal way now. Formal definition: Improve over task T, with respect to performance measure P, based on experience E.
Difference between Supervised and Unsupervised Learning - BYJUS The accuracy of results produced are less in unsupervised learning models. Training the model to predict output when a new data is provided is the objective of Supervised Learning. ... Number of classes are known in Supervised Learning. ... Some popular examples of supervised machine learning algorithms are: Linear regression for regression ... What is Supervised Learning? - tutorialspoint.com Supervised learning, one of the most used methods in ML, takes both training data (also called data samples) and its associated output (also called labels or responses) during the training process. The major goal of supervised learning methods is to learn the association between input training data and their labels. CS 229 - Supervised Learning Cheatsheet - Stanford University Probably Approximately Correct (PAC) PAC is a framework under which numerous results on learning theory were proved, and has the following set of assumptions: the training and testing sets follow the same distribution; the training examples are drawn independently Real-Life Examples of Supervised Learning and Unsupervised Learning ... Unsupervised Learning When we don't have labels for the inputs, our model should be able to find patterns and regularities in the input that are unknown for us, humans. We need to estimate which associations occur more often than others and how they are related.
In supervised learning, class labels of the training samples are ... Expert-verified answer scouteo In supervised learning, class labels of the training samples are "known." The correct answer is "known." The other options for the question were "unknown," "partially known," and "doesn't matter." It cannot be "unknown," because training samples must be known.
Semi-Supervised Learning: Techniques & Examples [2022] - V7Labs Semi-supervised learning is a broad category of machine learning that uses labeled data to ground predictions, and unlabeled data to learn the shape of the larger data distribution. Practitioners can achieve strong results with fractions of the labeled data, and as a result, can save valuable time and money.
ch8 - Data Mining: Concepts and Techniques (3rd ed.)... supervised learning (i.e., the learning of the classifier is "supervised" in that it is told to which class each training tuple belongs). unsupervised learning (or clustering), in which the class label of each training tuple is not known, and the number or set of classes to be learned may not be known in advance.
Supervised learning - Wikipedia Supervised learning (SL) is the machine learning task of learning a function that maps an input to an output based on example input-output pairs. [1] It infers a function from labeled training data consisting of a set of training examples. [2] In supervised learning, each example is a pair consisting of an input object (typically a vector) and ...
ML | Types of Learning - Supervised Learning - GeeksforGeeks Supervised learning is when the model is getting trained on a labelled dataset. A labelled dataset is one that has both input and output parameters. In this type of learning both training and validation, datasets are labelled as shown in the figures below. Both the above figures have labelled data set as follows:
An in-depth guide to supervised machine learning classification Supervised Learning. In supervised learning, algorithms learn from labeled data. After understanding the data, the algorithm determines which label should be given to new data by associating patterns to the unlabeled new data. Supervised learning can be divided into two categories: classification and regression.
What is Supervised Learning? | IBM What is supervised learning? Supervised learning, also known as supervised machine learning, is a subcategory of machine learning and artificial intelligence. It is defined by its use of labeled datasets to train algorithms that to classify data or predict outcomes accurately.
Unstructured Data Classification.txt - In Supervised learning, class ... in supervised learning, class labels of the training samples are known select pre-processing techniques from the options all the options a classifer that can compute using numeric as well as categorical values is random forest classifier classification where each data is mapped to more than one class is called multi-class classification tf-idf is …
Supervised vs Unsupervised Learning Explained - Seldon Examples of supervised learning classification. A classification problem in machine learning is when a model is used to classify whether data belongs to a known group or object class. Models will assign a class label to the data it processes, which is learned by the algorithm through training on labelled training data.
Unsupervised Learning and Data Clustering | by Sanatan Mishra | Towards ... K-means is one of the simplest unsupervised learning algorithms that solves the well known clustering problem. The procedure follows a simple and easy way to classify a given data set through a certain number of clusters (assume k clusters) fixed a priori. The main idea is to define k centres, one for each cluster.
What is Supervised Learning? | TIBCO Software Supervised learning solves known problems and uses a labeled data set to train an algorithm to perform specific tasks. It uses models to predict known outcomes such as "What is the color of the image?" "How many people are in the image?" "What factors are driving fraud or product defects?" etc.
Prototypical Classifier for Robust Class-Imbalanced Learning In this paper, we address both training set biases simultaneously. As shown in Fig. 1a, it is known that the classifier directly learned on class-imbalanced data is biased towards head classes [8, 32] which results in poor generalization on tail classes.Moreover, using sample loss/confidence produced by biased classifiers fails to detect label noise, because both clean and noisy samples of ...
Supervised vs Unsupervised Learning: Difference Between Them - Guru99 Unsupervised learning is computationally complex. Use of Data. Supervised learning model uses training data to learn a link between the input and the outputs. Unsupervised learning does not use output data. Accuracy of Results. Highly accurate and trustworthy method. Less accurate and trustworthy method.
Post a Comment for "42 in supervised learning class labels of the training samples are known"